A single-cell resolved cell-cell communication model explains lineage commitment in hematopoiesis
Megan K. Franke, Adam L. MacLean
Read the preprint
One of the first papers I read in biology, over a decade ago — during my transition into the field from undergraduate physics — was:
Forcing cells to change lineages. In this classic review, Graf and Enver capture the new reality of cell reprogramming: cell fates are not fixed; with the right combination of transcription factors, a fibroblast can become a myocyte, or even a pluripotent stem cell. A fascination with cell fates and cell fate malleability took hold within me then, and has quietly continued to shape the problems I work on ever since.
More recently,
Megan Franke, a PhD student in our lab, came to me with an idea: a new modeling framework to combine gene regulatory networks with cell-cell communication at the single-cell level. This addressed what we saw as a rather urgent unmet need: models of gene regulatory dynamics generally dismiss any dynamic signaling processes originating outside the cell. Yet, we know: all cells exist in a noisy and tumultuous extracellular signaling milieu.
To test the idea, we chose a canonical gene regulatory network from the literature: the GATA1-PU.1 mutual inhibition loop that controls myeloid lineage specification in hematopoiesis. Specifically, it controls the bifurcation of multipotent progenitor cells into one of two fates (granulocyte-monocyte or megakaryocyte-erythroid committed cells). This model, specified by nonlinear ordinary differential equations (ODEs), permits bistability. We tightly constrained the model so that the steady states were fixed for all parameters, i.e. all cells must commit to either the GATA1-high or the PU.1-high state, according to a single bifurcation diagram (shown below). In this highly controlled system, the essential determinant of cell fate becomes cell-cell communication, which we modeled with a Poisson process. We considered both consensus signals passed between cells ("be like me") and dissensus signals ("be unlike me").
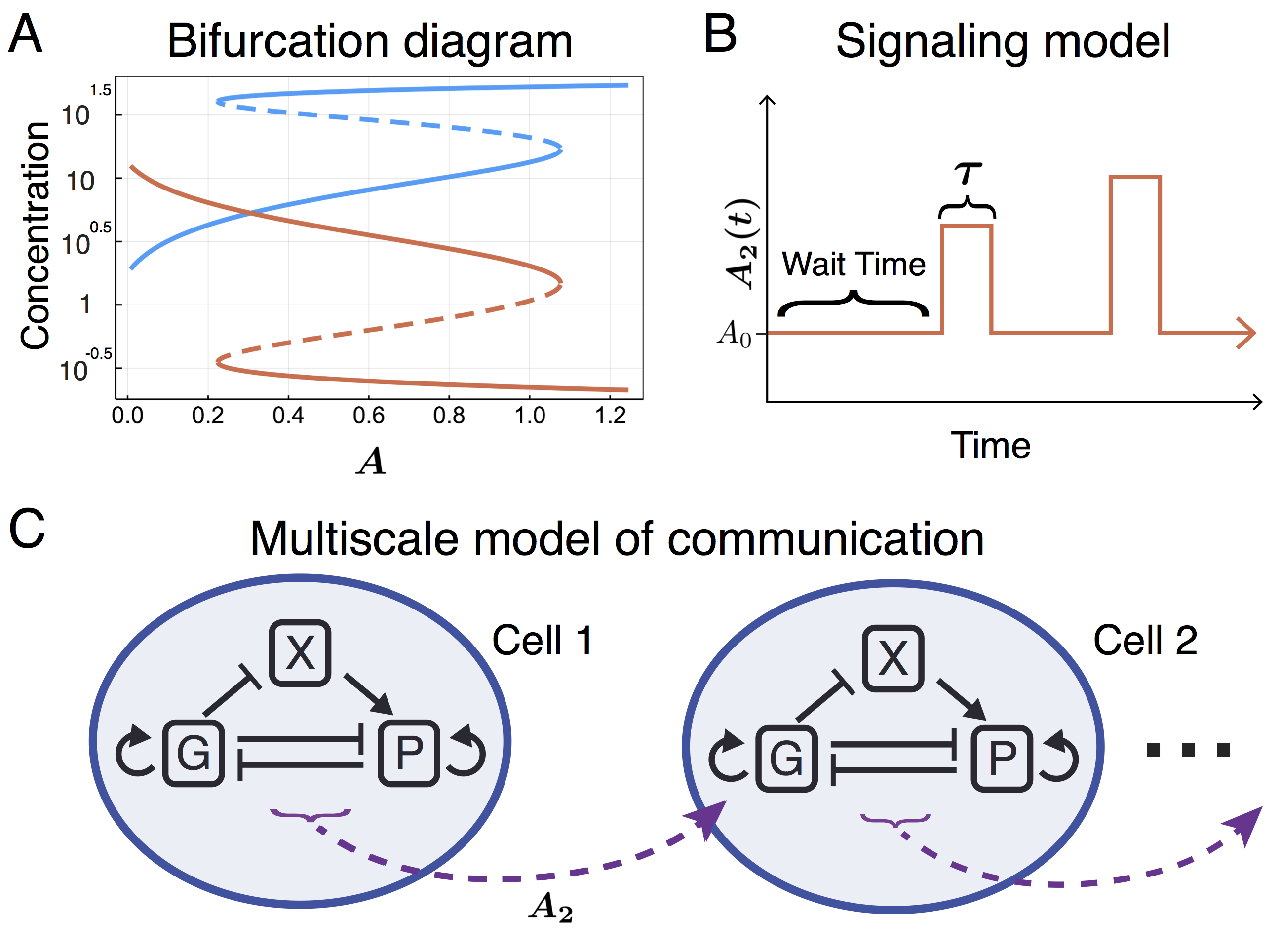
We studied a wide range of cell-cell communication topologies, and found that across this range even very subtle effects due to cell-cell communication resulted in dramatic shifts in cell fate distributions. We also discovered that the model resolved controversies arising in the literature. Most prominently, that of whether or not stochastic fluctuations in protein levels are sufficient to initiate myeloid lineage cell fate choices. Previous models assumed that stochastic protein fluctuations can initiate lineage commitment, i.e. different initial conditions of the ODE system will lead to different cell fate outcomes. However,
Hoppe et al. showed — through a series of elegant live imaging experiments – that this could not be the case, but rather that “these transcription factors are only executing and reinforcing lineage choice once made.” There must be an external factor responsible for the initial symmetry breaking. Our results reveal that cell-cell communication could be exactly this missing factor.
This work was made possible in part thanks to the fast and flexible tools offered for systems biology modeling in the
Julia programming language, particularly
DifferentialEquations.jl. This allowed us to create a new framework for multiscale simulations: whereas previous works have modeled either the gene regulatory dynamics or cell-cell communication in high-resolution, none have (to our knowledge) modeled both simultaneously. Previously, simplifying assumptions were made either regarding the cell-internal dynamics (treating it as a black box) or the cell-cell communication dynamics (treating it as a diffusive process). In order to resolve cell-cell communication between individual cells as well as cell-internal GRN dynamics, we developed a hybrid deterministic-stochastic model capable of simulating the dynamics of cell-cell communication within and between single cells. From a stochastic processes perspective, the model closely resembles a piecewise deterministic Markov process.
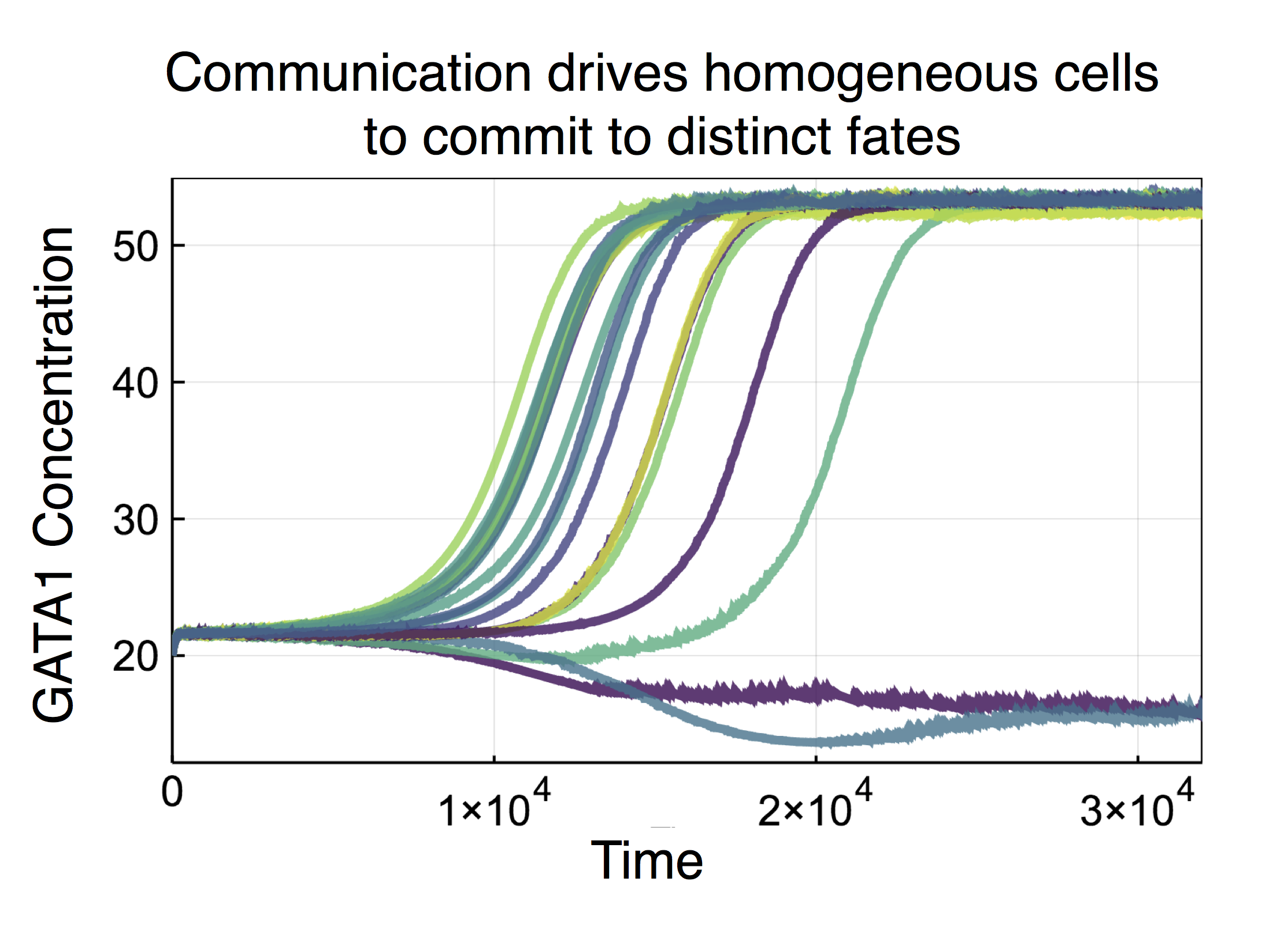
In the paper we also analyze the effects of noise in signaling between cells. We considered both extrinsic noise (resulting from signal distortion in the extracellular milieu) and intrinsic noise (resulting from signal distortion during signal transduction inside the cell following a ligand-receptor binding event). We found that both sources of noise acted to increase the variability of cell fates, with extrinsic noise producing much larger effects than intrinsic noise (in agreement with previous analyses in a
very different system). Noise also propagates down chains of communicating cells, leading to an increase in their cooperativity in cell fate decision-making. Strikingly, we also found that the addition of noise led to a shift in the likelihood of committing to a particular fate, i.e. the presence of noise affects not only the variance – but also the mean – of the distribution: noise alters the cell fate decision-making boundaries!
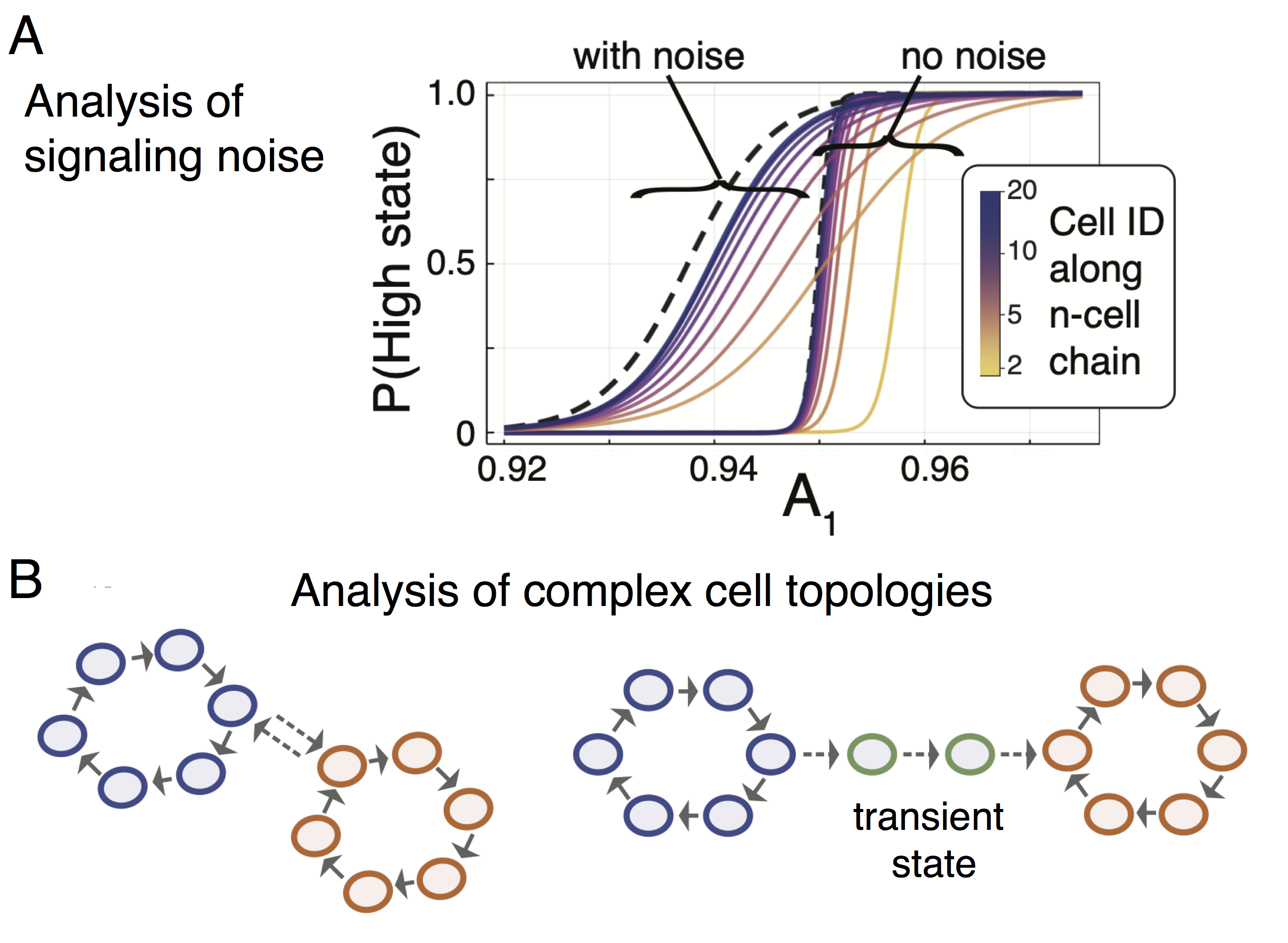
So, what about cancer? The cell fate system studied here is of healthy stem cell differentiation, but the implications for cancer are evident. Cell-cell communication plays an essential role in defining cancer cell fates, and thus clinical outcomes. In addition to (probably) the most obvious/important: CAR T cell—tumor cell interactions, cell-cell communication dictates a wide range of cancer cell fate decisions, from epithelial-to-mesenchymal transition induced by TGF-beta, to ovarian cancer stem cell "stemness," maintained by cell-cell communication via a specific WNT ligand (WNT5B)
produced from M2 macrophages.
We anticipate this framework for modeling cell-cell communication to be widely applicable to a broad range of systems in cancer. All that is required for its application is knowledge of a gene regulatory network that controls a cancer cell phenotype of interest, and information about how signals passed via cell-cell communication affect the GRN dynamics. With these two ingredients, small or large topologies of interacting cells can be simulated and the emergent effects on cell phenotypes studied. Of particular relevance here may more the complex topologies of interacting cells, e.g. driven through a transient state characterizing malignant transformation (see figure above). Overall, we hope this work encourages the community to think more deeply about how external signals affect gene regulatory dynamics, and the possible impact: forcing cells to change lineages by cell-cell communication.
The paper is available
here and the all the associated code is available is available
here.