How to use transcriptomic data for game-theoretic modeling of treatment-induced resistance in cancer cells? A case study in patient-derived glioblastoma organoids
Weronika Gąska, Christer Lohk, Maikel Verduin, Christopher Hubert, Marc Vooijs, Rachel Cavill, Kateřina Staňková
Read the preprint
We decided we would like to understand how to get beyond models of cancer and its treatment that are fitted with volumetric information only
3,4, to be able to predict the effect of anti-cancer treatments and improve therapy through game-theoretic modeling. The results of this effort are in our preprint
1.
That is why we paired up with Marc Vooijs and his team (
www.maastrolab.com), who can grow patient-derived glioblastoma organoids (PDOs) under irradiation
5. With organoids, where the total tumor cell population is kept constant, we can track how treatment impacts the cell type composition over time without needing invasive biopsies. This makes organoids an ideal testing ground for the game-theoretic models describing changes in the proportion of different cell types in time, such as replicator dynamics. The question we had was whether we can somehow, from all the transcriptomics data, figure out how different PDOs respond to two different doses of irradiation (4 and 10 Gy), in terms of changes to the proportions of different cell types in time. We got time-series data, but only 4 time points, including initial composition in treated and untreated organoids. As this was our first attempt to use transcriptomics in our modeling, we were looking for methodologies for translating this data into inputs for our game-theoretic models.
So how do we disentangle this data to extract what different cell types are present in this mixture and how their proportions change over time? It is sort of a smoothie problem:
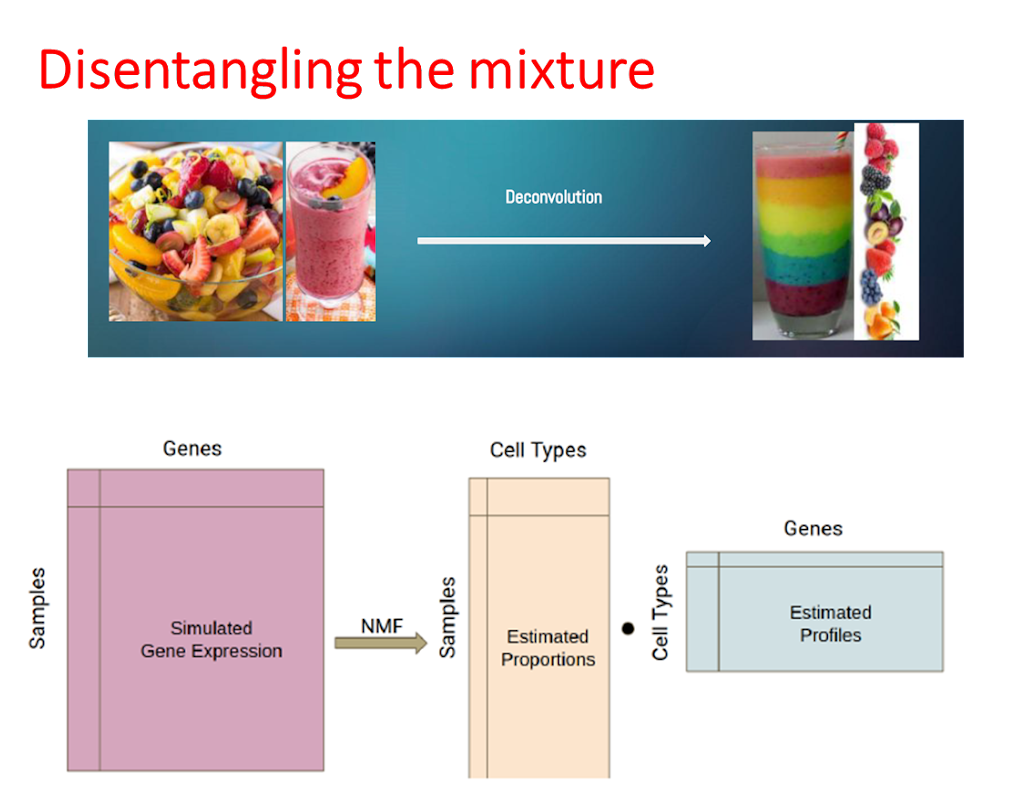
We have a series of smoothies mixed with different, yet unknown fruits. And the question is whether we can figure out how many fruits are in, what their characteristics are, and what the proportion of each fruit is in each smoothie. Now here, the smoothie is the organoid - each organoid contains different cell types which have different characteristics (like the different fruit), and each organoid has different proportions of these different cell types.
Furthermore, our smoothie is changing composition over time, in response to the treatment. At this point we had a choice, we could go into it completely blind, using unsupervised methods, meaning that we do not presuppose what types of fruits there are, nor what each fruit looks like ahead of our analysis in an unsupervised deconvolution. Or we could take from a selection of fruits we might expect to see in our smoothies and use these profiles to define the fruits we were looking for, in a supervised deconvolution.
Back to our bulk data from organoids. We applied both the supervised and the unsupervised deconvolution methods to identify different cell types in the organoids. In the supervised case we used public single cell data, to give us profiles of the cell types we would expect to see in the tumors. And then we tracked how the proportions of these cell types changed over time. In the case of unsupervised method, we first used the TCGA (The Cancer Genome Atlas) dataset to identify cell types, as this was a much larger dataset than our organoids. In this case nothing is assumed about the proportions nor the profiles of the cell types in advance of the analysis.
For the unsupervised methods we ran into issues surrounding the different types of cells that are present in real patient, compared to those present in the organoids. This meant that several of the cell types we identified from the patient data, couldn’t really be detected in the organoids (the algorithm gave them a low proportion in every sample). After looking at the genes which characterized these types, we could see they were probably things such as immune cells which we wouldn’t expect to see in the organoids.
Figure 1: Population dynamics of mesenchymal-like and cycling cells in patient-derived glioma organoids under two divergent conditions. Hypoxia-independent cells are MES-like 1 and Hypoxia-depedent cells are MES-like 2 in Neftel’s scheme
We had more luck with the supervised analysis. Using single cell data from other publications, especially
2, we were able to make artificial mixtures in silico, with known proportions of each cell type, and then train a neural network to predict the proportions from the data. Using this neural network on the real data we could see how the proportions of cycling cancer cells changed in response to treatment (Fig. 1). More than 96% of all organoids (at all timepoints) appear to consist of mesenchymal-like cells while the remaining 4% (or less) belong to other cell types, which have constantly low proportions. So we focused on just modeling the mesenchymal-like cells.
Figure 2: Fitted replicator dynamics equations vs. the measurements and ESS (horizontal line). First point (not fitted) indicates the measurement before the therapy is applied.
We fit the obtained proportions to replicator equations model (Fig. 2). One could say: “Mission accomplished”, but many questions remain, such as these ones:
- Can we validate that these predictions are correct through more time series data?
- Does irradiation lead to stabilization of the proportion of different cell types in all patients?
- What is data that we can use for our game-theoretic models better?
Currently we are looking into single-cell sequencing and images. With single-cell sequencing, we can skip the deconvolution step, but experience so far has shown us that clustering single-cell measurements into ‘cell types’, particularly recognizable ones which can be easily interpreted, is not as straightforward as one would imagine. With imaging data we can also get spatial information about how different cell types tend to cluster round or avoid other cell types, which can both be used to estimate the fitness matrix in our replicator equations, or, if it changes in response to treatment, may be a measure of resistance. We could then model this with spatial models.
- Can we combine the estimated proportions through organoids with volumetric measurements in real patients (similarly to 3 and 4), to fit and validate models of eco-evolutionary dynamics?
Answering these questions is ahead of us, thus lots of fun to come!
References
- Gąska, W. et al (2022) How to use transcriptomic data for game-theoretic modeling of treatment-induced resistance in cancer cells? A case study in patient-derived glioblastoma organoids? bioRxiv 2022.01.26.477755. https://doi.org/10.1101/2022.01.26.477755
- Neftel, C. et al. (2019) An Integrative Model of Cellular States, Plasticity, and Genetics for Glioblastoma. Cell, 178(4), 835–849.e21. https://doi.org/10.1016/j.cell.2019.06.024
- Ghaffari Laleh, N. et al. (2022) Classical mathematical models for prediction of response to chemotherapy and immunotherapy. PLoS Comput Biol 18(2): e1009822. https://doi.org/10.1371/journal.pcbi.1009822
- Martinez, V.A. et al. (2022) Improving mathematical models of cancer by including resistance to therapy: a study in non-small cell lung cancer. bioRxiv 2021.10.29.466444. https://doi.org/10.1101/2021.10.29.466444
- Hubert, C. G et al. (2016). A Three-Dimensional Organoid Culture System Derived from Human Glioblastomas Recapitulates the Hypoxic Gradients and Cancer Stem Cell Heterogeneity of Tumors Found In Vivo. Cancer research, 76(8), 2465–2477. https://doi.org/10.1158/0008-5472.CAN-15-2402