The concept of adaptive therapy is exciting to many of us in mathematical oncology for at least three reasons: 1) this approach to therapy is based on evolutionary concepts, 2) this approach to therapy is informed by mathematical modeling, and 3) this approach has been tested in clinical trials. 2021 saw the release of important data from the trial, including Gleason scores, metastatic locations, initial PSA values, and cumulative dose of both the standard of care cohort and its adaptive counterpart
1. It's likely that we may never seen a randomized clinical trial in this setting, due to the fact that standard of care for metastatic prostate cancer has changed with early abiraterone administration in the castrate sensitive setting
1. (The trial administered abiraterone adaptively in the castrate-resistant setting.)
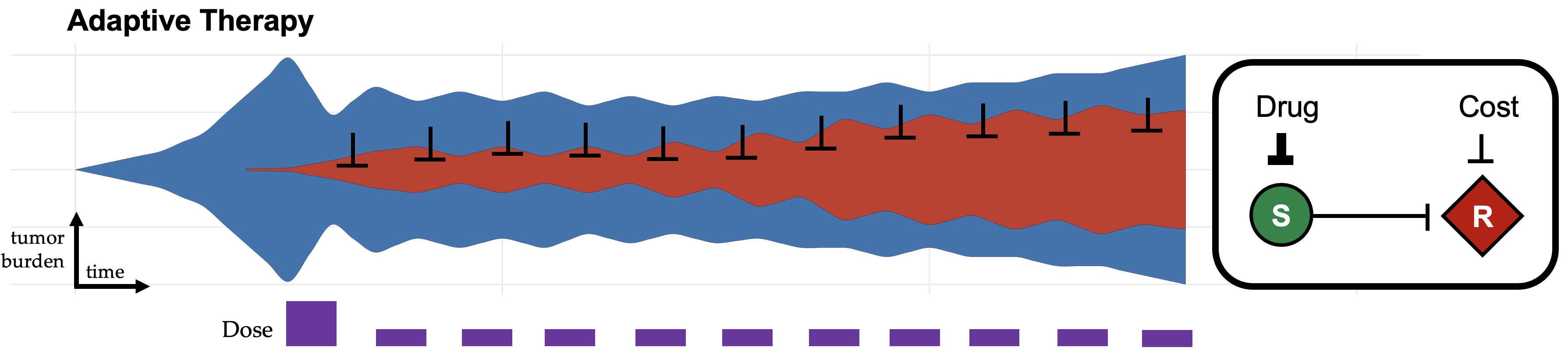
The paper also notes that the trial has inspired deeper investigations into the mathematical models of adaptive therapy to investigate the clinical circumstances when adaptive approaches are likely to be effective. We'll explore some of these approaches that were published in 2021, below (listed in no particular order).
Published in 2021
Darwinian Approaches for Cancer Treatment: Benefits of Mathematical Modeling
Sophia Belkhir, Frederic Thomas, Benjamin Roche
Read the paper
With the original adaptive therapy paper amassing over 600 citations
2, it's high time for a review paper summing up all the advances in this space. The Roche review, published in David Basanta's special issue "Understanding the Evolutionary Dynamics and Ecology of Cancer in Treatment Resistance," answers the call
3. The review first gathers the reasons for failure of convential treatment strategies (e.g. competitive release) before reviewing the principles behind adaptive therapy (e.g. competitive suppression of resistance). Then, the authors produce a table collating many of the various mathematical models published, with respective model type, cancer type, number of cell types, parameters of interest, and predicted outcomes. By far the most common modeling methods are Lotka-Volterra style and agent-based methods.
Do mechanisms matter? Comparing cancer treatment strategies across
mathematical models and outcome objectives
Cassidy K. Buhler, Rebecca S. Terry, Kathryn G. Link, Frederick R. Adler
Read the paper
With so mathematical models published in this space, Fred Adler undertook the important task of collating many of these models into one unifying rubric and performing identical analysis across varied assumption on mechanisms: 1) competition with healthy cells, 2) inclusion of resource dynamics, 3) an immune response, and 4) an Allee effect
4. The authors tested how a comprehensive set of intermittent and adaptive therapies balance two types of treatment failure: resistance threshold or tumor burden threshold. I won't belabor more explanation, as Fred has already written an excellent "Behind the Paper" post,
here.
A theoretical analysis of tumour containment
Yannick Viossat & Robert Noble
Read the paper
Another mathematical tour de force in adaptive therapy was published in Nature Ecology & Evolution by Rob Noble and Yannick Viossat, complete with a 31 (!) page supplemental material
5. The paper reads partly like a review of the field, along with great consolidation of recent results into a cohesive mathematical analysis. It's an instant classic, must read.
Predicting patient-specific response to adaptive therapy in metastatic castration-resistant prostate cancer using prostate-specific antigen dynamics
Renee Brady-Nicholls, Jingsong Zhang, Tian Zhang, Andrew Z. Wang, Robert Butler, Robert A. Gatenby, Heiko Enderling
Read the paper
Given the clinical translatability of adaptive therapy approaches, it's of high importance to quantify the ability of mathematical models to predict response to therapy.
Renee Brady-Nicholls does this using her stem cell model of abiraterone resistance in this work, published in Neoplasia
6. View the corresponding cover artwork for the manuscript
here!
Optimizing Adaptive Therapy Based on the Reachability to Tumor Resistant Subpopulation
Jiali Wang, Yixuan Zhang, Xiaoquan Liu, Haochen Liu
Read the paper
This research investigate the trade-off between adaptive and maximum tolerable dose therapy (MTD), where adaptive approaches are favored when resistance is non-aggressive, and MTD is favored for tumors with aggressive resistant subpopulations
7. The authors introduce a "restore index" to guide therapy choice. Intriguingly, the experimental system which informs the modeling does NOT have a cost of resistance, which is often explicitly assumed in many modeling approaches. It's nice to see this group publish several important pieces in this field this year, including our next feature:
Is the Fixed Periodic Treatment Effective for the Tumor System without Complete Information?
Jiali Wang, Yixuan Zhang, Xiaoquan Liu, Haochen Liu
Read the paper
This might be my (totally biased) pick for my favorite of 2021. The authors take an experimental system derived from an EGFR-mutant and a KRAS-mutant cell line, parameterize a Lotka-Volterra style model using in vitro data in order to determine optimal treatment schedules, and subsequently test treatment strategies in vivo
8. One of the many reasons I enjoyed the paper is the fact the authors attempted our "evolutionary cycles" concept in an experimental system (driving a tumor into periodic cycles where tumor composition roughly matches initial pre-treatment composition
9). Incredibly, this approach can be achieved with fixed (i.e. non-adaptive) treatment regimens!
Are Adaptive Chemotherapy Schedules Robust? A Three-Strategy Stochastic Evolutionary Game Theory Model
Rajvir Dua, Yongqian Ma, Paul K. Newton
Read the paper
Speaking of evolutionary cycles, my Ph.D. advisor (Paul Newton, Univ. Southern California) continued his investigations of the viability of evolutionary cycles with a focus on stochastic dynamics
10. The papers offers analysis of possible evolutionary strategies that minimize the likelihood of saturation of any subclonal cell type within the tumor.
Preprints published in 2021
Where would science be without preprints? Let's highlight a few preprints from 2021.
Spatial structure impacts adaptive therapy by shaping intra-tumoral competition
Maximilian A. R. Strobl, Jill Gallaher, Jeffrey West, Mark Robertson-Tessi, Philip K. Maini, Alexander R. A. Anderson
Read the preprint
In this preprint,
Maximilian Strobl introduces an extension of last year's
Cancer Research publication11 which explicitly accounts for the role of spatial interactions
12. The preprint ends by fitting the model to the dynamics of 67 prostate cancer patients on intermittent androgen deprivation therapy.
The impact of the spatial heterogeneity of resistant cells and fibroblasts on treatment response
Masud M A, Jae-Young Kim, Cheol-Ho Pan, Eunjung Kim
Read the preprint
Following the trend of spatial agent-based models, this next preprint introduces a new cell type to the modeling framework: fibroblasts
13. The preprint provides a comprehensive investigation of the role of cell migration, carrying capacity, resistance distribution, and fibroblast microenvironmental factors on outcomes of adaptive treatment.
The impact of the spatial heterogeneity of resistant cells and fibroblasts on treatment response
Masud M A, Jae-Young Kim, Cheol-Ho Pan, Eunjung Kim
Read the preprint
The last preprint we'll look at is an investigation of the biomarker used in adaptive prostate treatment scheduling: prostate-specific antigen (PSA). This biomarker has been used clinically to determine treatment decision-making, but mathematical models typically assume that all cell types within a tumor contribute to the PSA biomarker in equal portion. The authors model current clinical protocols under a variety of assumptions about PSA in order to assess the efficacy of adaptive therapy when various cell types produce more PSA than others.
Looking forward in 2022
I would be remiss not to close out this post without looking forward to the yet unanswered questions surrounding adaptive therapy. I hope 2022 will bring more mathematical models to investigate the following topics:
- What diseases are ideal targets for adaptive therapy?
- What is the role of plasticity in adaptive treatment?
- What are the best practices to extending adaptive therapy to multiple drugs?
- Can mathematical modeling drive personalized treatment scheduling in the clinic?
In my opinion, these questions are fertile ground for math onco in 2022. What else?
References
- Zhang, J., Cunningham, J.J., Brown, J.S. et al. Response to Mistry. Nat Commun 12, 329 (2021). https://doi.org/10.1038/s41467-020-20175-3
- Gatenby, Robert A., et al. "Adaptive therapy." Cancer Research 69.11 (2009): 4894-4903.
- Belkhir, Sophia, Frederic Thomas, and Benjamin Roche. "Darwinian approaches for cancer treatment: benefits of mathematical modeling." Cancers 13.17 (2021): 4448.
- Buhler, Cassidy K., et al. "Do mechanisms matter? Comparing cancer treatment strategies across mathematical models and outcome objectives." Mathematical Biosciences and Engineering 18.5 (2021): 6305-6327.
- Viossat, Yannick, and Robert Noble. "A theoretical analysis of tumour containment." Nature Ecology & Evolution 5.6 (2021): 826-835.
- Brady-Nicholls, Renee, et al. "Predicting patient-specific response to adaptive therapy in metastatic castration-resistant prostate cancer using prostate-specific antigen dynamics." Neoplasia 23.9 (2021): 851-858.
- Wang, Jiali, et al. "Optimizing adaptive therapy based on the reachability to tumor resistant subpopulation." Cancers 13.21 (2021): 5262.
- Wang, Jiali, et al. "Is the Fixed Periodic Treatment Effective for the Tumor System without Complete Information?." Cancer Management and Research 13 (2021): 8915.
- West, Jeffrey, et al. "Towards multidrug adaptive therapy." Cancer Research 80.7 (2020): 1578-1589.
- Dua, Rajvir, Yongqian Ma, and Paul K. Newton. "Are adaptive chemotherapy schedules robust? A three-strategy stochastic evolutionary game theory model." Cancers 13.12 (2021): 2880.
- Strobl, Maximilian AR, et al. "Turnover modulates the need for a cost of resistance in adaptive therapy." Cancer Research 81.4 (2021): 1135-1147.
- Strobl, Maximilian AR, et al. "Spatial structure impacts adaptive therapy by shaping intra-tumoral competition." bioRxiv (2021): 2020-11.
- Masud, M. A., et al. "The impact of the spatial heterogeneity of resistant cells and fibroblasts on treatment response." bioRxiv (2021).